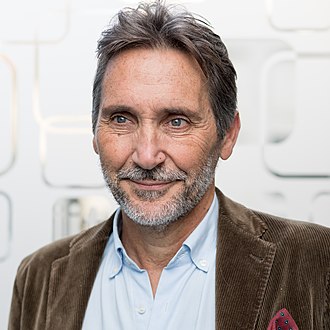
Antonio Bicchi
Professor
Department of Information Engineering
University of Pisa
Senior Researcher
Istituto Italiano di Tecnologia in Genoa
Italy
Title: Towards Human-Robot Symbiosis
Abstract: Recent research advancements in the field of robotics have made it possible not only to have machines that approach or beat the computational intelligence of humans, but are also capable of ever more natural motion and exploit the “physical” intelligence embodied in their structure. Informed by neuroscientific models of human behavior in interaction with the physical world, new robots can safely touch humans and the environment to physically act on it. New sensing and display tools make it possible for other senses than just vision to share information on the world between a robot and a human. The union of such technologies, together with a deeper understanding of how to interface humans and machines, is enabling a new relationship between humans and robots, that is really more an integration than an interaction in the classical sense. We will consider examples of partial integration, as in prosthetics and rehabilitation, augmentation with supernumerary limbs, augmentation with exoskeletons, and robotic avatars, with the robot executing the human’s intended
actions and the human perceiving the context of his/her actions and their consequences.
Bio: Antonio Bicchi is Professor of Robotics at the University of Pisa, and Senior Scientist at the Italian Institute of Technology in Genoa. He graduated from the University of Bologna in 1988 and was a postdoc scholar at M.I.T. Artificial Intelligence lab in 1988–1990. He teaches Robotics and Control Systems in the Department of Information Engineering (DII) of the University of Pisa. He leads the Robotics Group at the Research Center “E. Piaggio” of the University of Pisa since 1990, where he was Director from 2003 to 2012. He is the head of the SoftRobotics Lab for Human Cooperation and Rehabilitation at IIT in Genoa. Since 2013 he serves ad Adjunct Professor at the School of Biological and Health Systems Engineering of Arizona State University.
His main research interests are in Robotics, Haptics, and Control Systems. He has published more than 400 papers on international journals, books, and refereed conferences. He was Editor-in-Chief of the IEEE Robotics and Automation Letters, which he founded in 2015. He has organized and chaired the first WorldHaptics Conference (2005). He was Editor of the book series “Springer Briefs on Control, Automation and Robotics,” and has served as an Editor for the Int.l J. Robotics Research, IEEE Trans. on Robotics and Automation, IEEE Trans. Automation Science and Engineering, and IEEE RAS Magazine. He has been Program Chair of Robotics: Science and Systems (RSS) 2019, IEEE Int. Conf. Robotics and Automation (ICRA’16), the Int. Symposium on Robotics Research (ISRR’ 2015) , Hybrid Systems: Computation and Control (HSCC 2007). In 2005 he co-founded and chaired of the first conference in the WorlHaptics series. He was Editor in Chief of the Conference Editorial Board for the IEEE Robotics and Automation Society (RAS), Vice President for Publications (2013-2014), for Membership (2006-2007), and Distinguished Lecturer (2004-2006) of IEEE RAS. He served as the President of the Italian Association or Researchers in Automatic Control (2012-2013). He is a co-founder and serves as President of the Italian Institute of Robotics and Intelligent Machines (I-RIM) since 2019, He is the recipient of several awards and honors. For his research on human and robot hands the European Research Council awarded him with four grants, including an Advanced Grant in 2012 and a Synergy Grant in 2018. Antonio Bicchi is a Fellow of IEEE since 2005.
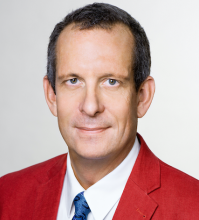
Martin Buss
Professor
Department of Electrical and Computer Engineering
Technische Universität München
Germany
Title: (TBA)
Abstract: (TBA)
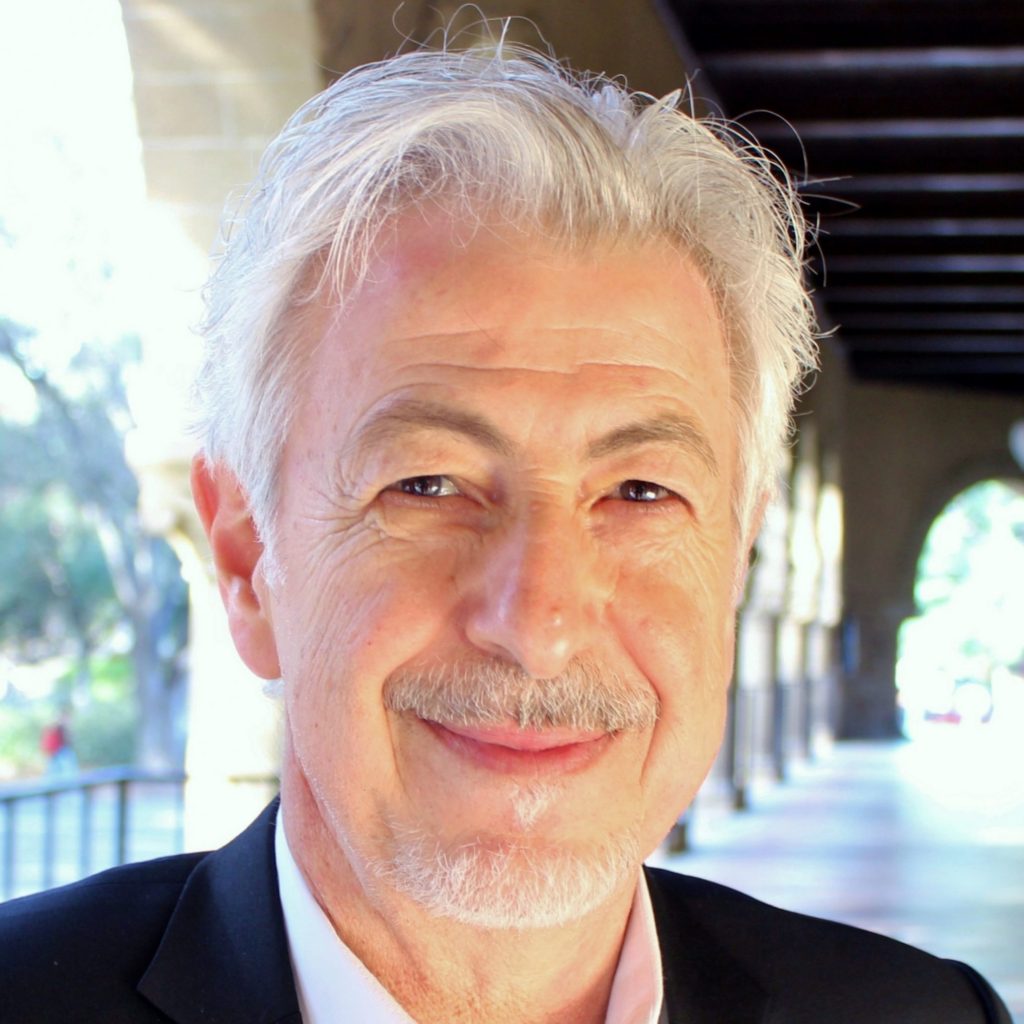
Oussama Khatib
Professor
Computer Science Department
Stanford University
USA
Title: The Era of Human-Robot Collaboration
Abstract: (TBA)
Bio: Oussama Khatib received his PhD from Sup’Aero, Toulouse, France, in 1980. He is Professor of Computer Science and Director of the Robotics Laboratory at Stanford University. His research focuses on methodologies and technologies in human-centered robotics, haptic interactions, artificial intelligence, human motion synthesis and animation. He is President of the International Foundation of Robotics Research (IFRR) and a Fellow of the Institute of Electrical and Electronic Engineers (IEEE). He is Editor of the Springer Tracts in Advanced Robotics (STAR) series, and the Springer Handbook of Robotics, awarded the American Publishers Award for Excellence in Physical Sciences and Mathematics. He is recipient of the IEEE Robotics and Automation (IEEE/RAS) Pioneering Award (for his fundamental contributions in robotics research, visionary leadership and life-long commitment to the field), the IEEE/RAS George Saridis Leadership Award, the Distinguished Service Award, the Japan Robot Association (JARA) Award, the Rudolf Kalman Award, and the IEEE Technical Field Award. In 2018, Professor Khatib was elected to the National Academy of Engineering.
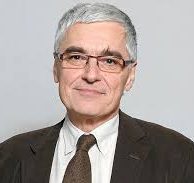
Jean-Paul Laumond
Directeur de Recherche, CNRS
Département d’Informatique
École normale supérieure
France
Title: Robotics: the science of motion
Abstract: Robots are moving machines that are subject to the laws of physics. This statement may appear obvious. However, it is easier today to make a machine playing chess than to make a bipedal robot walking! Robotics research requires to explore the computational foundations of the relationship between the machine and its environment. The expected actions a robot has to perform are expressed in the physical world. Robots —like any living being— access the physical space through its body space made of its sensory space and its motor space. The degree of autonomy of a system depends on its ability to translate the action expressed in the physical space into its sensory-motor space. This statement has been grounded by mathematician Henri Poincaré. It will be illustrated by the study of locomotion for humanoid robots. Walking seems to be an every day simple task. However, robotics research shows that the task is not as easy as it appears at first glance. By trying to solve the problem for humanoids, roboticist contributes not only to design new machines,
but also to explore open questions in biomechanics and neurophysiology. Robotics is not only a matter of engineering but also a matter of science: the science of motion.
Bio: Jean-Paul Laumond, IEEE Fellow, is a roboticist. He is Directeur de Recherche at CNRS. Previously with LAAS-CNRS in Toulouse, he joined in 2019 the research unit DIENS affiliated to ENS, CNRS and INRIA (team Willow) in Paris. His research is devoted to robot motion planning and control. In 2001 and 2002 he created and managed Kineo CAM, a spin-off company from LAAS-CNRS to develop and market motion planning technology in the domain of Product Life Management. Siemens acquired Kineo CAM in 2012. In 2006, he launched the research team Gepetto dedicated to Human Motion studies along three perspectives: artificial motion for humanoid robots, virtual motion for digital actors and mannequins, and natural motions of human beings. From 2014 to 2018, he managed the project Actanthrope (ERC-ADG 340050) devoted to the computational foundations of anthropomorphic action.He teaches Robotics at Ecole Normale Supérieure in Paris. He has published more than 150 papers in international journals and conferences in Robotics, Computer Science, Automatic Control and in Neurosciences. He has been the 2011-2012 recipient of the Chaire Innovation technologique Liliane Bettencourt at Collège de France in Paris. He is the 2016 recipient
of the IEEE Inaba Technical Award for Innovation Leading to Production. He is a member of the French Academy of Technologies and of the French Academy of Sciences.
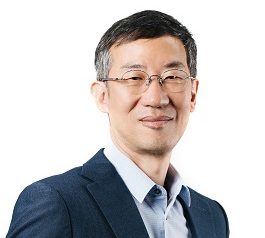
Frank Park
Professor
School of Mechanical and Aerospace Engineering
Seoul National University
Korea
Title: To Learn or Not to Learn: Robot Modeling in the Age of Machine Learning
Abstract: Models for the kinematics and dynamics of robots are essential in applications ranging from robot control and motion optimization to collision detection and interactive skill learning. Physics-based simulations based on kinodynamic models are also increasingly being used to generate training data for robot learning algorithms. It goes without saying, however, that a model is only as good as the accuracy of its identified parameters. Although it has been traditional to decouple kinematic and dynamic model identification and to view the two problems as being “solved”, this is far from the case, particularly for high-dof robots equipped with low-cost noisy sensors. These challenges explain in part the recent trend toward constructing approximate kinodynamic robot models via nonparametric learning methods, but such models are narrowly limited in their effectiveness.
In this talk we argue that many of these challenges can be mitigated through a unified geometric approach. Building on the seminal and foundational work of Y. Nakamura and his group on robot model identification, we exploit the underlying geometry of robot kinodynamic models—masses are positive and inertia tensors are positive-definite, for example—and frame unified kinodynamic model identification as an optimization problem on a certain matrix Lie group. Efficient gradient-based algorithms can efficiently and robustly identify both the kinematic and dynamic parameters, even for high-dof robots with noisy sensor measurements.
This geometric perspective can in fact be generalized to a wide class of problems in machine learning that eventually reduce to an optimization, e.g., manifold learning, autoencoder training. We show that these and related problems can in fact be formulated as generalizations of the notion of manipulability, a concept pioneered by T. Yoshikawa and Y. Nakamura in the 1980s that has found wide applicability in many diverse areas beyond robotics.
In the latter part of the talk we argue that machine learning methods are most effective when augmented to a kinodynamic model, to compensate for unmodelled dynamics effects like elasticity and friction. This point of view is justified with a detailed experimental case study of the AMBIDEX tendon-driven robot. (NOTE: This talk describes results of the PhD research of Dr. Taeyoon Lee and Jaewoon Kwon (forthcoming) and Dr. Cheongjae Jang, with contributions
from Naver Labs).
Bio: Frank C. Park received the B.S. degree in electrical engineering from MIT in 1985, and the Ph.D. degree in applied mathematics from Harvard in 1991. After joining the faculty of the University of California Irvine in 1991, since 1995 he has been a Professor of Mechanical and Aerospace Engineering with Seoul National University. He is fellow of the IEEE, has been an IEEE Robotics and Automation Society Distinguished Lecturer, and held adjunct faculty positions with the NYU Courant Institute, the Interactive Computing Department at Georgia Tech, and the HKUST Robotics Institute in Hong Kong. His research interests include robot mechanics, planning and control, vision and image processing, mathematical data science, and related areas of applied mathematics. He is a former Editor-in-Chief for the IEEE Transactions on Robotics, developer of the EDX course Robot Mechanics and Control I-II, and coauthor (with Kevin Lynch) of the textbook Modern Robotics: Mechanics, Planning, and Control (Cambridge University Press, 2017). He is incoming president of the IEEE Robotics and Automation Society for 2022–2023.
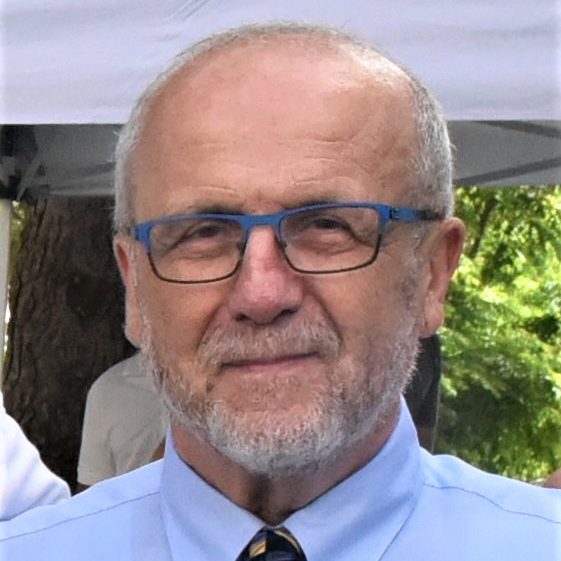
Zvi Shiller
Professor
Department of Mechanical Engineering and Mechatronics
Ariel University
Israel
Title: (TBA)
Abstract: (TBA)
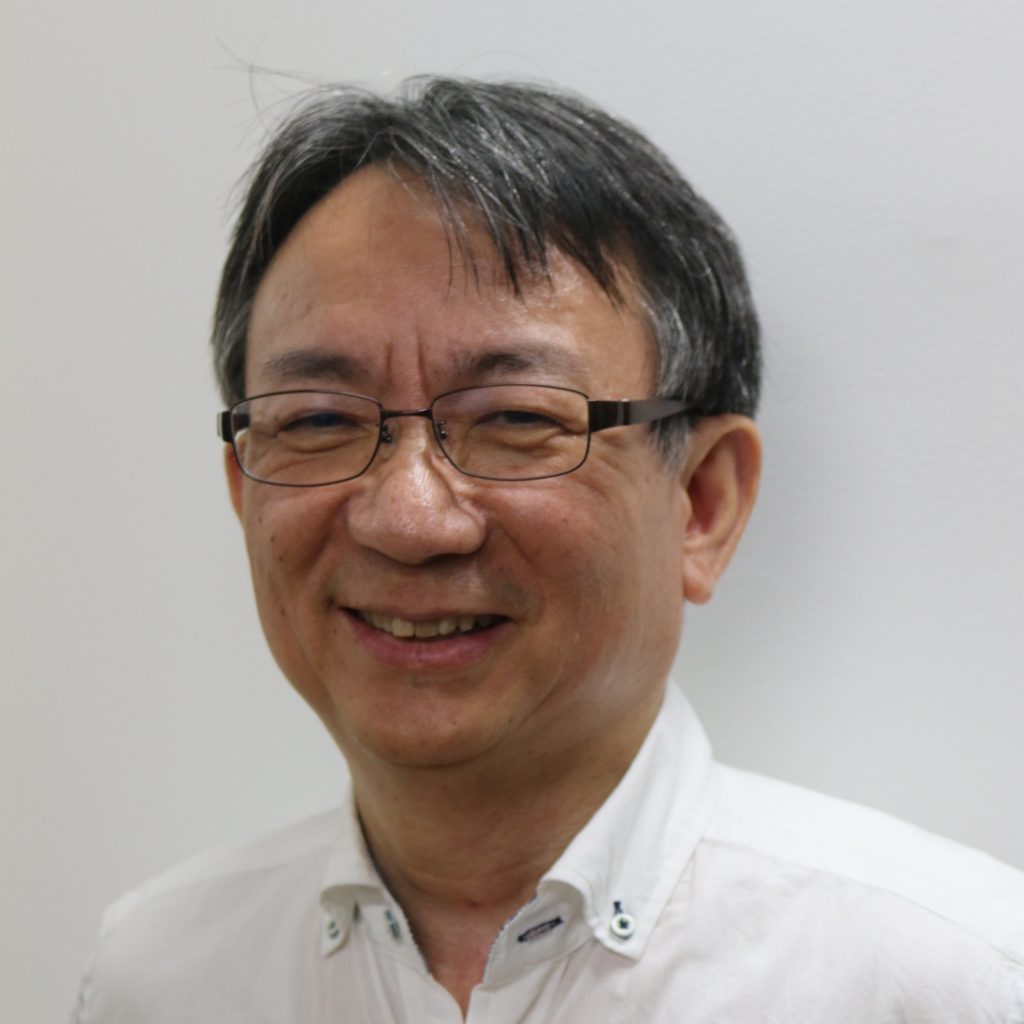
Toshiharu Sugie
Professor Emeritus, Kyoto University
Specially Appointed Professor
Graduate School of Engineering
Osaka University
Japan
Title: Kernel methods for nonparametric modeling and data-driven controller tuning
Abstract: Kernel methods, which play an important role in machine leaning, have attracted a lot of attention in the area of system identification recently. Once an appropriate kernel function is chosen, it is possible to obtain a fairly accurate finite impulse response model of the target system based on the short I/O data only. First, we show that the kernel based identification is useful to update the feedforward compensation in model matching problem. Its effectiveness is demonstrated through an experiment of quadcopter position control. Second, using a Gaussian kernel, we try to tune PID controllers based on the experimental data. Its effectiveness is demonstrated through experiments of a nonlinear mechanical system.
Bio: received the B.E., M.E., and Ph.D. degrees in engineering from Kyoto University, Japan, in 1976, 1978 and 1985, respectively. From 1978 to 1980, he was a research member of Musashino Electric Communication Laboratory in NTT, Musashino, Japan. From 1984 to 1988, he was a research associate of Department of Mechanical Engineering, University of Osaka Prefecture, Osaka. From 1988 to 2019, he worked at Kyoto University, where he was a Professor of Department of Systems Science from 1997 to 2019. In 2019, he joined Osaka University, where he is currently a specially appointed professor at Komatsu MIRAI Construction Equipment Cooperative Research Center in Graduate School of Engineering. His research interests are in robust control, identification, and control application to mechanical systems. He was as an Associate Editor of several international journals, and served as an Editor for Automatica (2008-2017). He was President of the ISCIE (Institute of Systems, Control and Information Engineers, Japan) in 2011, and a Vice President of the SICE (Society of Instrument and Control Engineers, Japan) in 2014-2015. He is a Fellow of IEEE and of IFAC.